Physicists Reveal Muscles’ Molecular Secret to Balancing Strength and Stamina
The findings could have implications for robotics, prosthetics and energy efficient machines.
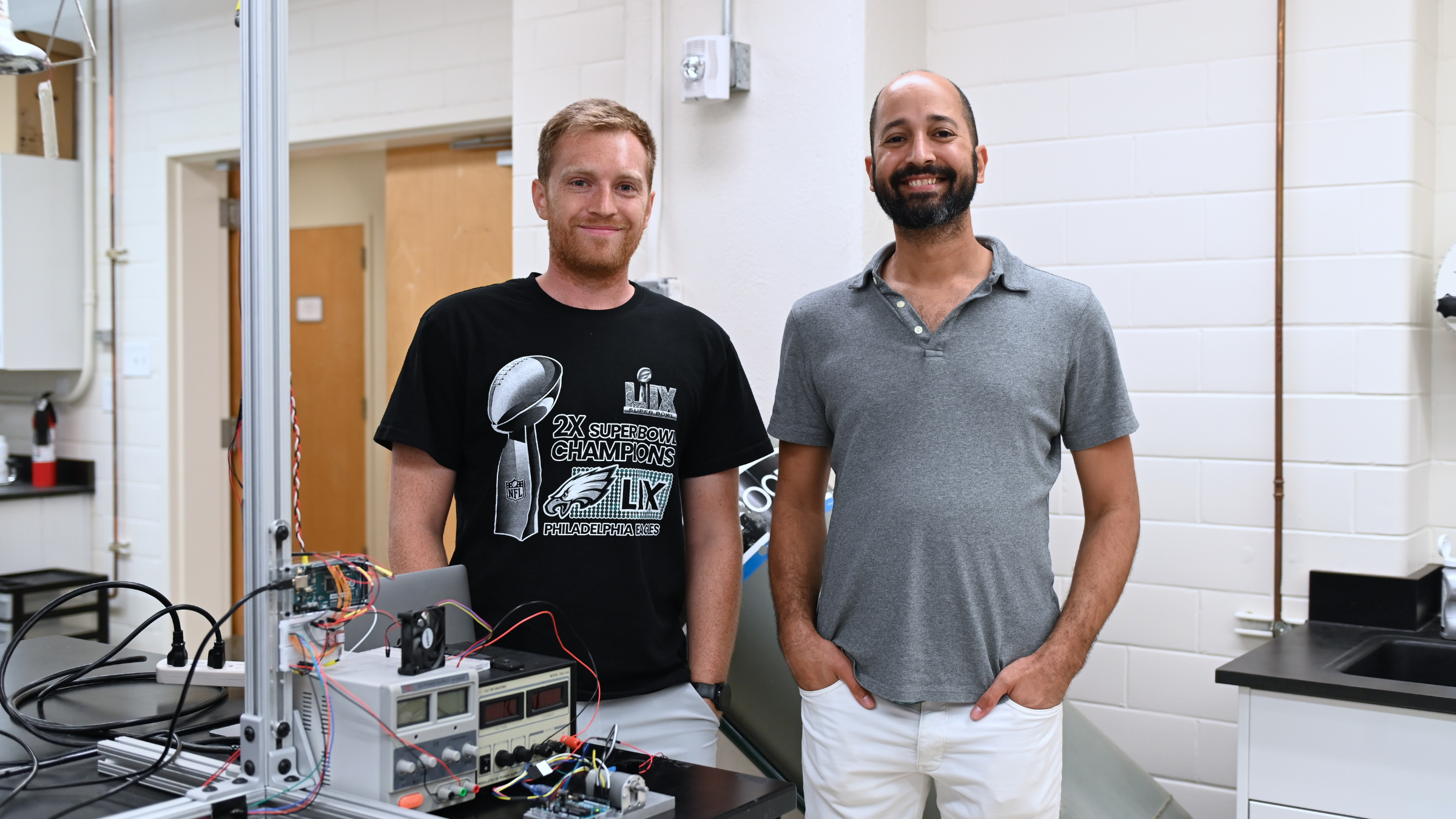
John McGrath and José Alvarado with HillBot, a robot designed to test parameters of muscle strength and efficiency. Photo by Yaqing Li, The University of Texas at Austin.
Muscles power everything from a cheetah’s sprint to a human heartbeat, but how do they balance power with efficiency? A new study from physicists at The University of Texas at Austin shows that this tradeoff is governed by a molecular tuning knob and that evolution has been optimizing this setting in identical patterns across species for millions of years. Their findings may help inform the design of energy-smart robots, prosthetics and bio-inspired machines.
The researchers focused on a parameter called α (alpha) from a nearly century-old equation called Hill’s muscle model, which describes how muscle force drops as contraction speed increases. While α has been widely reported in physiology studies, it has long been treated as a useful empirical constant, though the underlying mechanism was not clearly understood. The new study sheds light on that mechanism.
“This value has been staring us in the face for decades,” said José Alvarado, assistant professor of physics at UT Austin and senior author on the paper, out today in the journal Communications Biology, “But we hadn’t connected it to the actual behavior of molecular motors inside muscle.”
The researchers discovered that α directly relates to what happens between two proteins within muscle cells: namely, myosin motors, the proteins that drive muscle contraction, detach faster at higher speeds from actin filaments, the thread-like protein fibers beneath the plasma membrane of cells. This letting-go action saves energy but reduces force.
Using a new microscopic model, the team showed that changing α adjusts this balance. Lower α values deliver more power but burn more energy, while higher values conserve energy at the cost of strength. Most importantly, they found that the α value observed most often in nature, about 3.85, hits a sweet spot between the two.
To test their hypothesis, researchers compiled 136 measurements of α from across scientific literature, covering muscles from humans, birds, frogs, insects and even mollusks. Despite dramatic evolutionary differences, most muscles shared a similar α value.
“It’s kind of eerie,” said Jake McGrath, a physics graduate student at UT Austin. “Whether it’s a human arm or a bird wing, this same value shows up over and over. Nature seems to like muscles that are balanced, not too strong, not too slow.”
This convergence, the researchers argue, reflects evolutionary optimization. Muscles tuned near an α value of 3.85 are generalists, able to deliver good power and endurance without being extreme in either direction.
To take their work beyond theory, the team built a physical model of muscle they called HillBot, named after physiologist A.V. Hill, who originally derived α. The simple device uses a motor and control algorithms to mimic muscle contraction. By adjusting α in software, they could precisely test how different values affected performance.
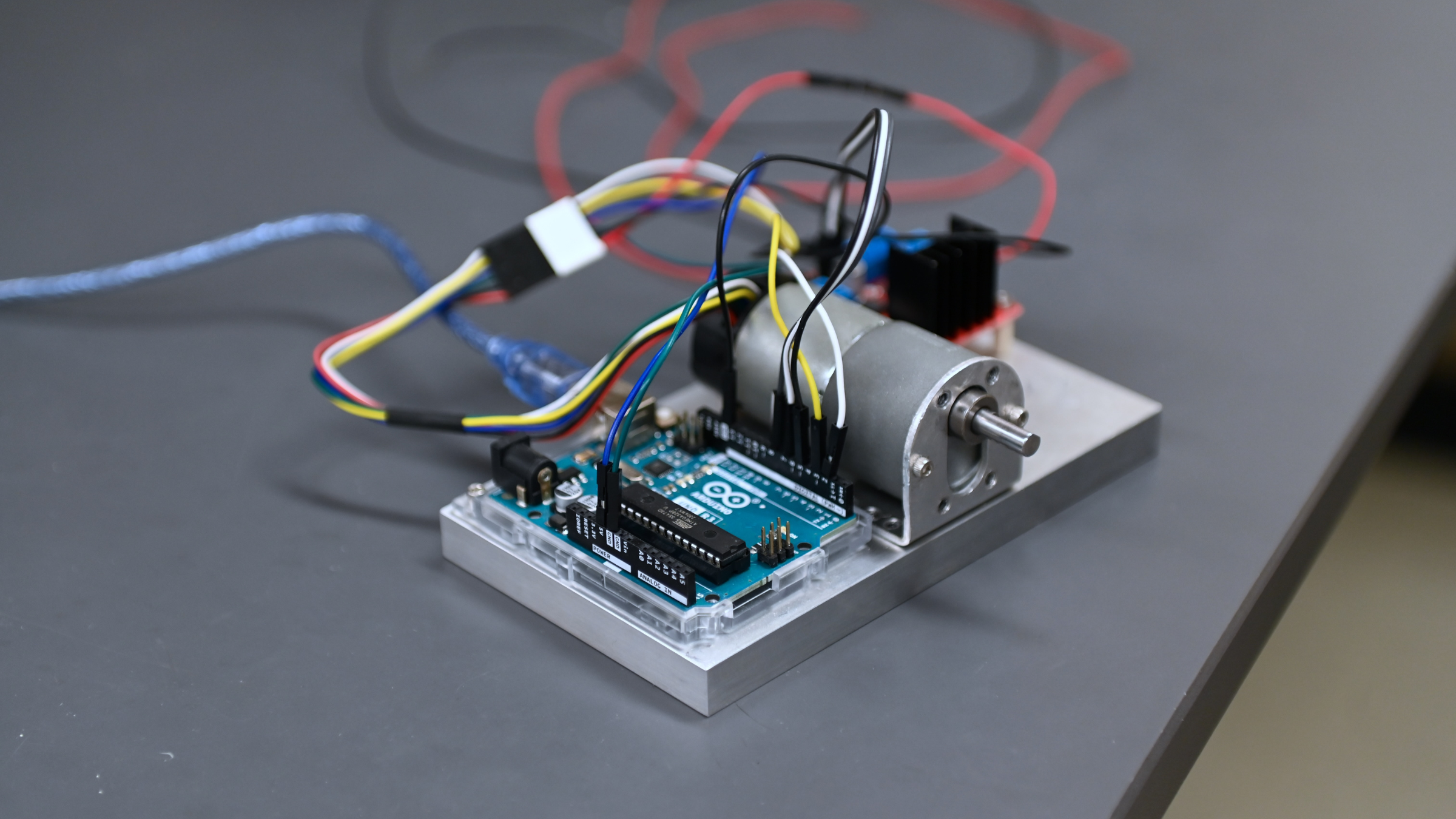
HillBot, a robot developed to test parameters of strength and efficiency in muscles. Photo by Yaqing Li, The University of Texas at Austin.
“In real muscle, it’s almost impossible to change just one variable like α,” McGrath said. “But with a robot, we can keep everything else constant and see exactly how efficiency and power respond.”
Remarkably, HillBot showed the same tradeoff pattern as the biological model. Higher α values led to more energy-efficient operation but lower power output.
“This was a key insight,” Alvarado said. “It showed us that it’s not just about chemistry—this tradeoff is baked into the physics of nonlinear systems.”
Because α can be tuned in real time in machines, the findings could help engineers design smarter, more adaptable devices.
“You could imagine a robot or prosthetic that switches modes,” McGrath said. “Power mode to lift something heavy. Efficiency mode to walk long distances. All controlled by software.”
The researchers also suggest that mimicking this biological nonlinearity could make robots safer and more energy-efficient—especially in devices that interact with humans.
“It turns out that muscle isn’t just a motor—it’s a motor that knows when to let go,” Alvarado said. “And now, we understand why that’s so powerful.”
Brian Kent and Colin Johnson of UT Austin were also authors on the paper. The research was conducted at UT Austin’s Center for Nonlinear Dynamics and the Weinberg Institute for Theoretical Physics. The research was funded by the National Science Foundation and the Gordon and Better Moore Foundation grant to the Kavli Institute for Theoretical Physics.