The Next 50 Years: An A.I. Designed to Make Life Better
Artificial intelligence is becoming more and more a part of our daily lives. But will AI have mostly positive or negative impacts on society?
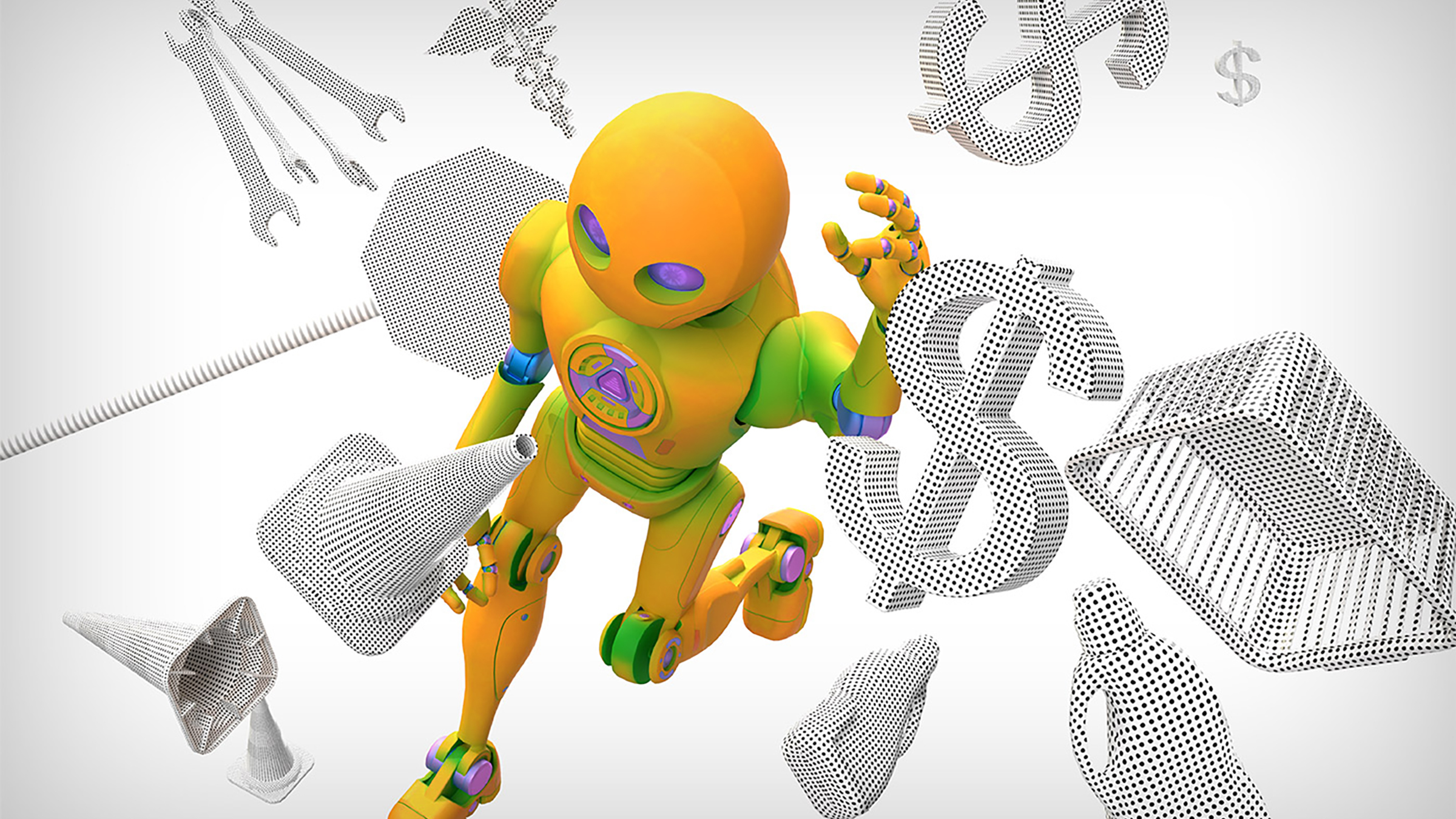
Some potential unintended consequences include home service robots that accidentally break your fine china, or systems that increase the gap between the haves and the have-nots. Peter Stone co-leads the Good Systems initiative at the University of Texas at Austin, which is trying to hash out guiding principles for building AI systems that are more likely to have a positive impact and fewer unintended consequences. He shares his team's vision for the future in this latest episode of our miniseries, The Next 50 Years.
Show Notes
Peter Stone also chaired the first technical report of the AI100 Study.
Music for today's show was produced by:
Podington Bear - https://www.podingtonbear.com/
Chuzausen - https://freemusicarchive.org/music/Chuzausen
TRANSCRIPT
MA: This is Point of Discovery, continuing our series on The Next 50 Years. What comes to mind when I say the words "artificial intelligence"? Do you think of Data, the android from Star Trek that's super intelligent, but always yearning to feel human emotions?
"But you don't have feelings, do you?"
"Not as such. However, even among humans, friendship is sometimes less an emotional response and more a sense of familiarity."
MA: Or do you think of more sinister machines, like HAL 9000 in 2001: A Space Odyssey?
"Open the pod bay doors, Hal."
"I'm sorry, Dave, I'm afraid I can't do that."
MA: Peter Stone is a computer scientist at The University of Texas at Austin who chaired the panel for the first-ever report by technology experts for the One Hundred Year Study of Artificial Intelligence, known as A.I. 100.
PS: Like almost any technology, most artificial intelligence technologies could be used for various purposes, ranging from good purposes to evil purposes. So the question is, it's not just a technical question, "Is an artificial intelligence technology good or bad?" It's also a social question. How is it used? What kind of regulations are put in place?
MA: Stone is looking at the future of A.I. in Texas as part of a university effort called Good Systems that has the goal to …
PS: … identify what are the principles of a system that's more likely to be used for good than for harm?
MA: Stone says the decisions we make, starting now, will determine whether we end up with A.I.s that benefit society. But right now, there are more questions than answers.
PS: What does it even mean to be good? What principles should we use when designing a system to maximize the chances they will be good? And these are sort of big, meaty questions that don't have easy answers.
MA: And you could ask, good for whom, right? So something that could be good for a government or a corporation might not be good for the average person?
PS: That's right. So good may be a function of the stakeholders. These are the questions that we have to wrestle with. Is "good" something that's just black and white? Or is it something that has a lot of nuance and what does it mean to be good for society? Who are the constituents of that society?
MA: For all of his expertise as an artificial intelligence researcher, Stone says this isn't a question only for people like him. One goal of the Good Systems effort is to bring people with diverse expertise together to grapple with these questions.
PS: It's something that we need to have input from experts in law and public policy and Information Systems and communications and many different directions.
MA: Other areas where AI can affect every-day life include things like transportation and domestic tasks. Stone has done a lot of research in these areas — from autonomous cars to smart intersections to "helper" robots. So I called him up on the phone for a follow-up conversation. One approach he's studying in transportation would evaluate options for improving traffic flow – for example, managed toll systems – in a way that balances the benefits and downsides for different stakeholders.
PS: You definitely want to be able to create incentive mechanisms that allow for the roadway systems to be used more efficiently overall. So what are the right metrics for that? Is it total system travel time? Is it the length of the longest path that anyone has to take?
MA: Depending on which metrics you maximize, some drivers might experience less time stuck in traffic, while others might experience more. The goal would be to determine the best outcome for the most people.
MA: Also as part of the Good Systems initiative, Stone is working with a colleague, Scott Niekum, on a project aimed at insuring that AI systems that are built and trained in a lab to tackle tasks under certain conditions will act the way they're intended to in the real world.
PS: So if, for instance, a robot is going to come out of the factory into my house, and be a home assistant robot that's going to help me unload my dishwasher and fold my laundry and things like that. Can we say that even though it's never been in my house before, we can be 99% sure that it won't fall down the stairs, or that we can be 95% sure that it won't knock over any delicate vases or something like that, can we characterize that as a performance bound before actually having run it?
MA: Once the AI has been trained, scientists create many different simulated environments and test to see how well it can do the task in those new environments.
PS: So, for example, if the robot has trained in you know, 1000 different houses, then from that you can say, well, you know, of these houses, this is the way stairs generally are detected and kitchens tend to be near the living rooms and you know, there's sort of this patterns that you learn from the past experience. And then you can use that to generate a whole bunch of feasible artificial houses, ones that the robot has never been in, but that are somehow similar to the ones that have been seen before. And then test your control algorithm in those.
MA: If the AI can perform the task correctly, say 99% of the time in these simulated houses, then designers will have high confidence that it will work correctly in the real world.
PS: When you're thinking from a Good Systems perspective, one of the things that you want to protect against is unintended consequences.
MA: AI systems could also lead to more serious unintended consequences: worsening income inequality, for example, if it were to enable a few big corporations and rich people to consolidate their wealth, while putting most of the rest of us out of work. It's something Stone and his colleagues examined in 2016 as part of that AI100 project.
PS: And so, we do need to be thinking about how can we keep society sustainable. We say this in the AI100 report that technology is increasing the size of the pie. So there should be a way to divide the pie such that everybody is better off. But there is a danger that it gets sliced thinner and thinner, and that many people are left behind. I think that's why social questions and economic questions, like "Should there be a universal basic income?" and things like that now get very tied up with artificial intelligence research. That's why we need people from different backgrounds as part of these conversations.
MA: With all of the potential downsides, what makes you hopeful that we will get it right?
PS: I don't know that there is a "getting it right." What makes me hopeful is that, I think, in general, technology, over time, is making the world a better place. If you asked me, would I rather have been born 100 years earlier than I was? I definitively would say, "No." I think that my life is better overall in today's world than it would have been any time in the past. I think, if you looked at humanity on average, that's probably the case that, on average, technology has helped humanity's quality of life go up.
MA: Next time on Point of Discovery, we'll explore a radical idea for understanding how life works – from the way healthy cells in your body process nutrients to the way cancerous cells grow out of control. Carlos Baiz envisions a computer model that is so detailed, it can keep track of every atom in a cell, and simulate the physical and chemical properties of all those atoms.
CB: So you would be able to have a movie … so to speak, an atomistic movie of how each atom is interacting with each other atom as the cell grows and reproduces. If there's a certain life process that you don't understand, you can go back to the movie right? You'd rewind, play it again, and then see how the molecules interact together and be able to piece together all the different parts.
MA: Baiz says it may take decades before computers are powerful enough to run such an advanced simulation. Stay tuned for the upcoming episode of our ongoing series, The Next 50 Years.
MA: Point of Discovery is a production of the University of Texas at Austin's College of Natural Sciences. Music for today's show is by Chuzausen and by Podington Bear. To read a transcript of this show — or find links to more podcasts and essays in The Next 50 Years series — visit us on our website at pointofdiscovery.org. If you like what you heard, be sure and tell your friends. We're available wherever you get your podcasts, including Apple Podcasts, Stitcher and Spotify. Our senior producer is Christine Sinatra. I'm your host and producer Marc Airhart. See you in the future!