Undergraduate Researchers Help Unlock Lessons of Machine Learning and AI
From large language models to brain-machine interfaces, students work with faculty on cutting-edge research.
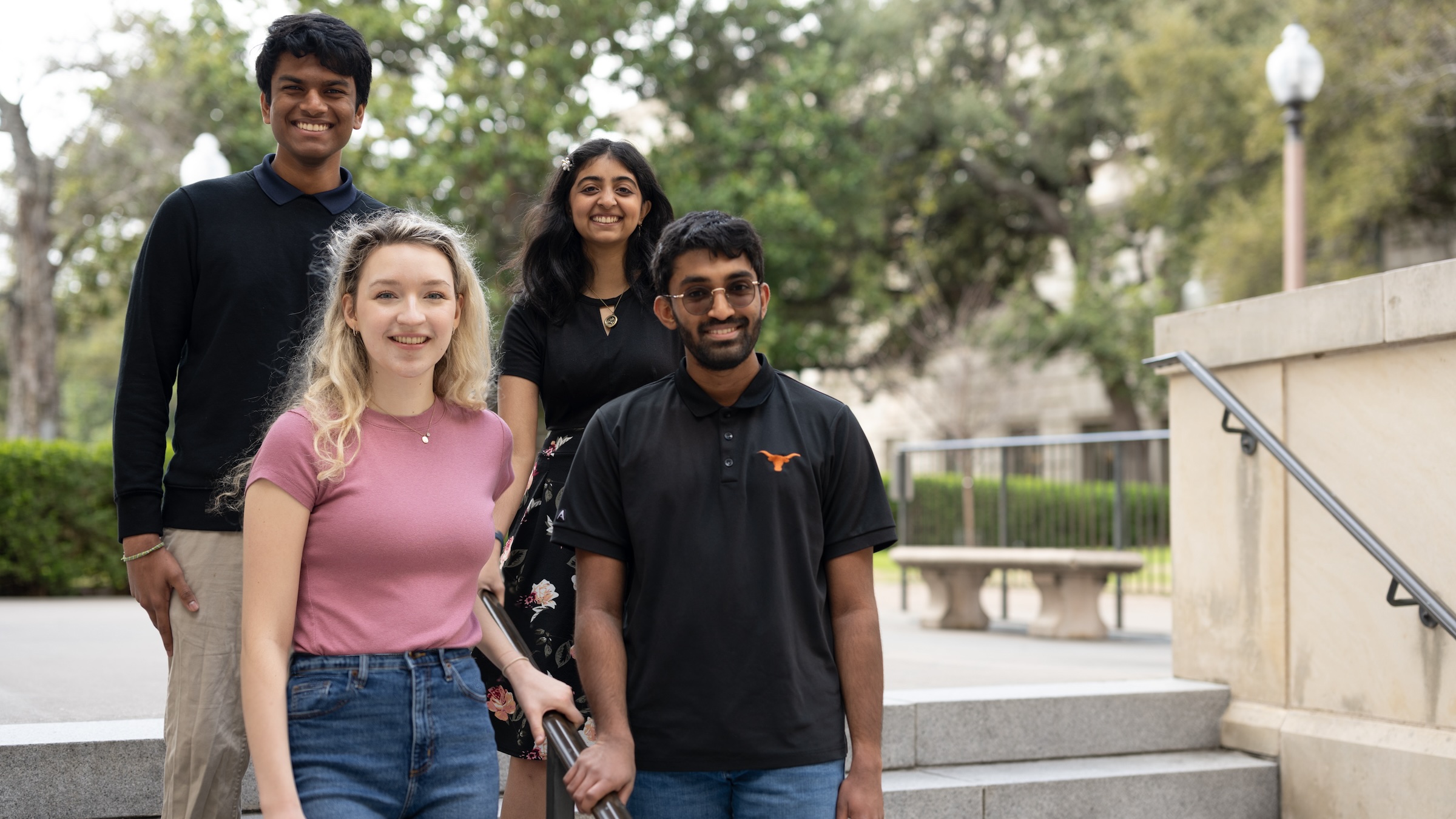
UT College of Natural Sciences undergraduates Prasann Singhal, Nihita Sarma, Shankar Padmanabhan and Jennifer Mickel are conducting research into AI and its applications.
Even before The University of Texas at Austin declared 2024 the Year of AI, artificial intelligence and machine learning had researchers across campus abuzz with activity. Undergraduates under the mentorship of professors in computer science and departments across campus are making their contributions in this fast-growing field.
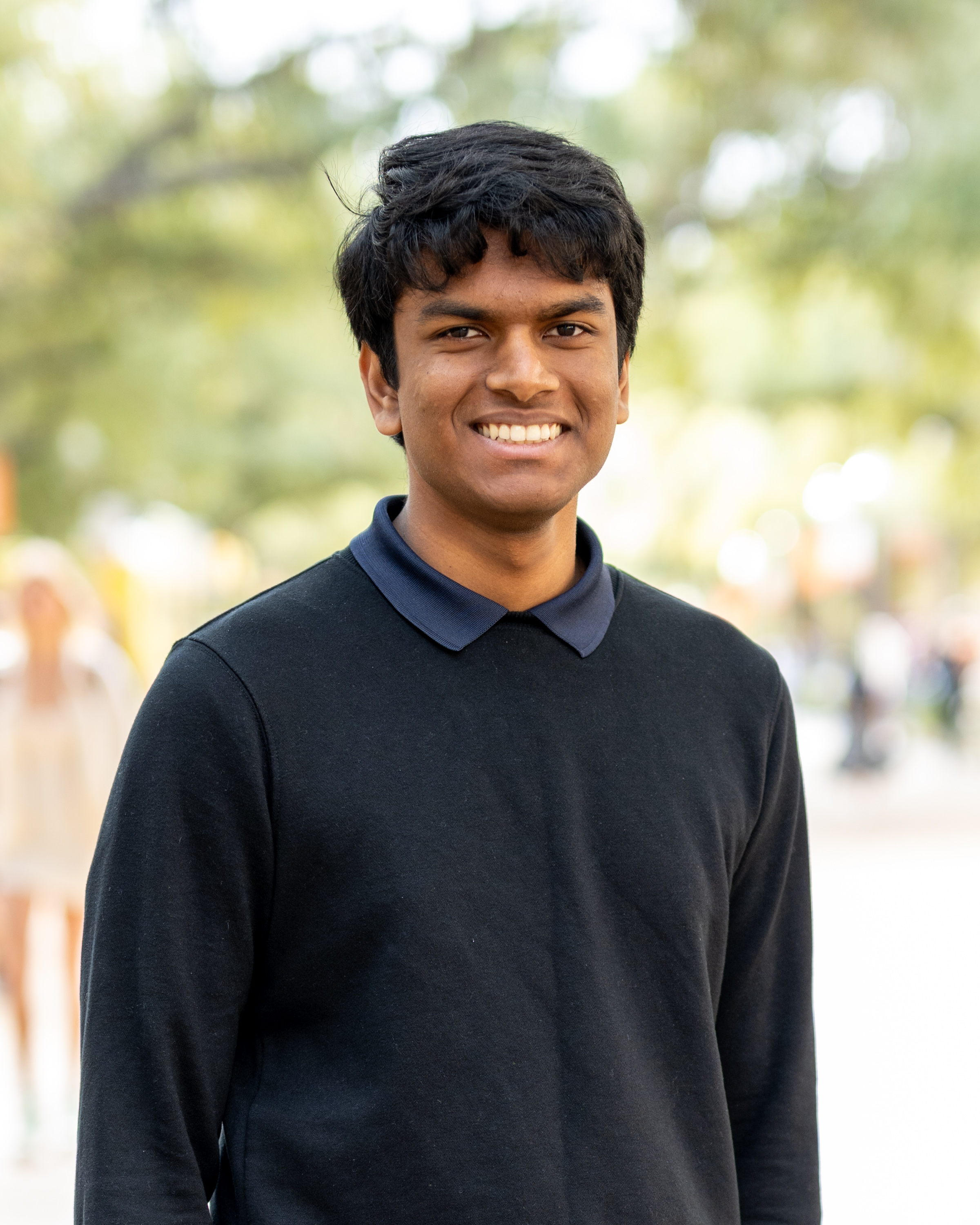
Prasann Singhal
Humanizing Responses
Prasann Singhal, linguistics and computer science junior, works under Greg Durrett, professor of computer science, to research the intersection of machine learning and human feedback. Using data and human preferences, Singhal’s methods seek to tweak machine-learning models to create output more aligned with what a human would want or expect. This alignment attempts to learn appropriate functions in a semi-supervised way without explicit instruction, Singhal said.
“A question like, ‘What are penguins?’ might produce an output that gives a scientific explanation of how penguins live their lives,” Singhal said. “That gets adjusted through technologies like ChatGPT to generate outputs that are more in line with what humans would expect and make them a little bit easier for the public to use.”
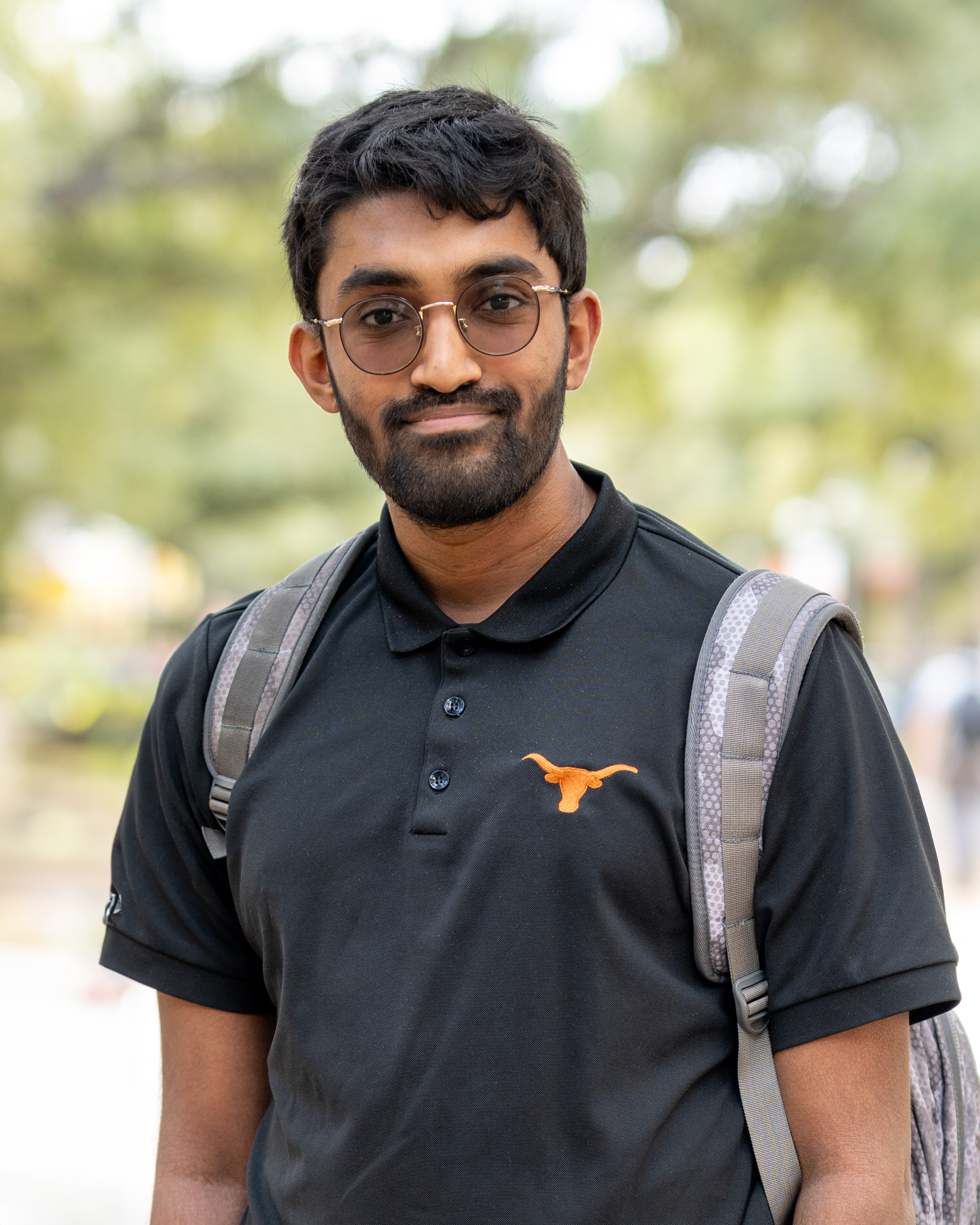
Shankar Padmanabhan
Knowing More
Mathematics senior Shankar Padmanabhan, researches another side of generative AI, model editing and continual learning, with computer science faculty member Eunsol Choi.
“Language models such as ChatGPT are usually trained on a fixed dataset (e.g, the internet before fall 2021),” said Padmanabhan. “As a consequence, they lack knowledge of events happening after this cutoff. The field of continual learning attempts to determine the best way to edit the knowledge base of these models without affecting their knowledge of prior events.”
Padmanabhan’s first paper discussed exploration of existing techniques, which he found were unable to make common-sense inferences. In a second paper, Padmanabhan found a method to fix discrepancies by placing a statement in context that outperformed prior models, he said. In December, Padmanabhan presented his research at the 2023 Neural Information Processing Systems conference, one of the biggest AI/machine learning conferences. Since then, Padmanabhan has been working on extending what he has learned to apply to information stored in languages other than English.
“In particular, if we inject some information in English into a language model, ideally it should be stored in a language-agnostic manner such that the model can use it even when it isn’t ‘talking’ in English,” Padmabhan explained.
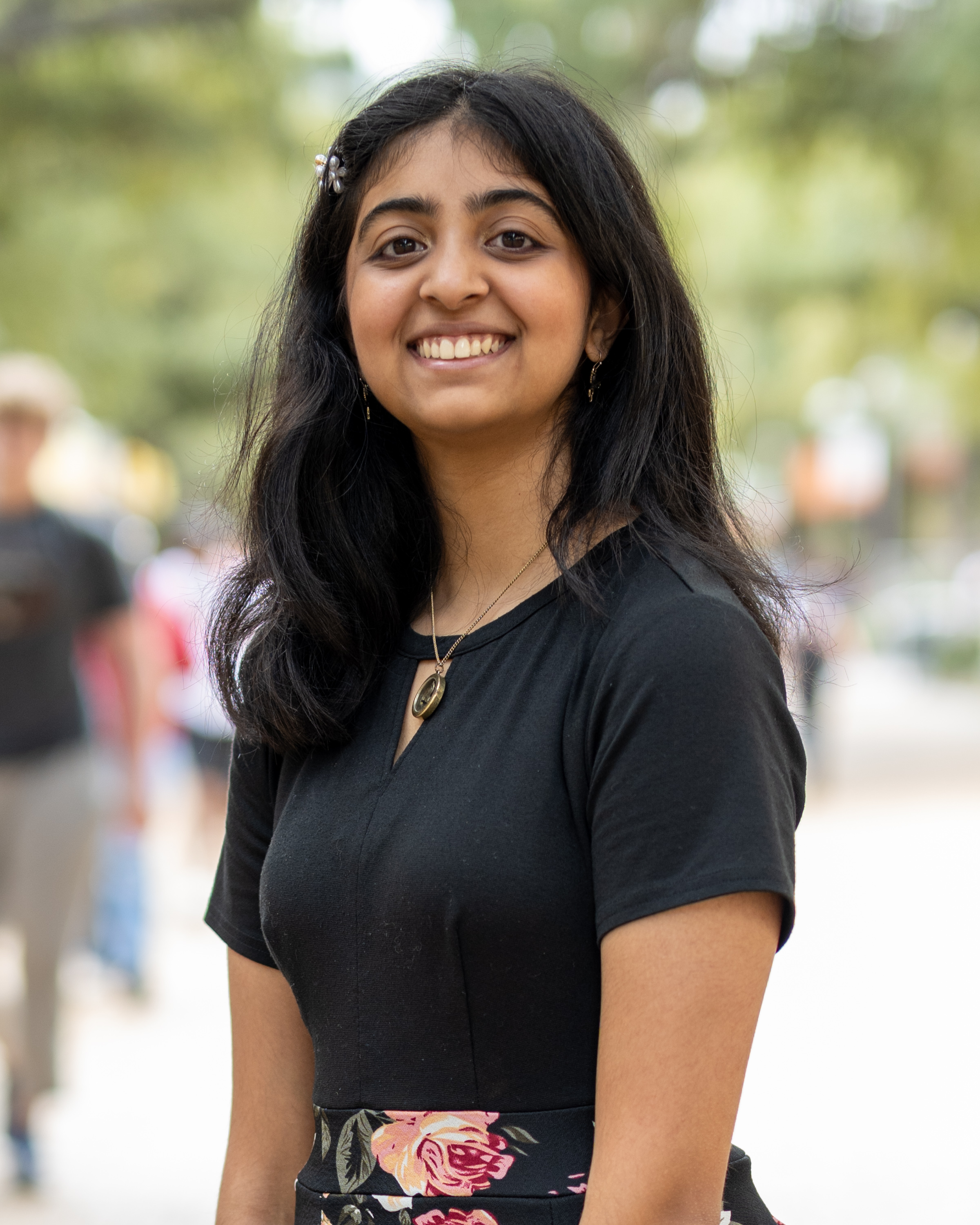
Nihita Sarma
Brain-Machine Interface
AI also intersects with language in other research areas. Nihita Sarma, a computer science third-year student and member of Dean’s Scholars and Turing Scholars, researches the intersection of neuroscience and machine learning to understand language in the brain, working with Alexander Huth, an assistant professor of computer science and neuroscience.
As research subjects listen to podcasts, they lie in an MRI machine and readings track their brain activity. These customized-to-the-subject readings are then used to train machine learning models called encoding models, and Sarma then passes them through decoding models.
“My research is taking those encodings and trying to backtrack and figure out based on this neural representation — based on the brain activity that was going on at that moment — what could the person inside the MRI machine possibly have been thinking or listening to at that moment?” Sarma said.
Along with gaining a better understanding of how language is represented in the brain, Sarma said the research has possible applications for a noninvasive communication tactic for people unable to speak or sign.
“We would be able to decode what they’re thinking or what they’re trying to say, and allow them to communicate with the outside world,” Sarma said.
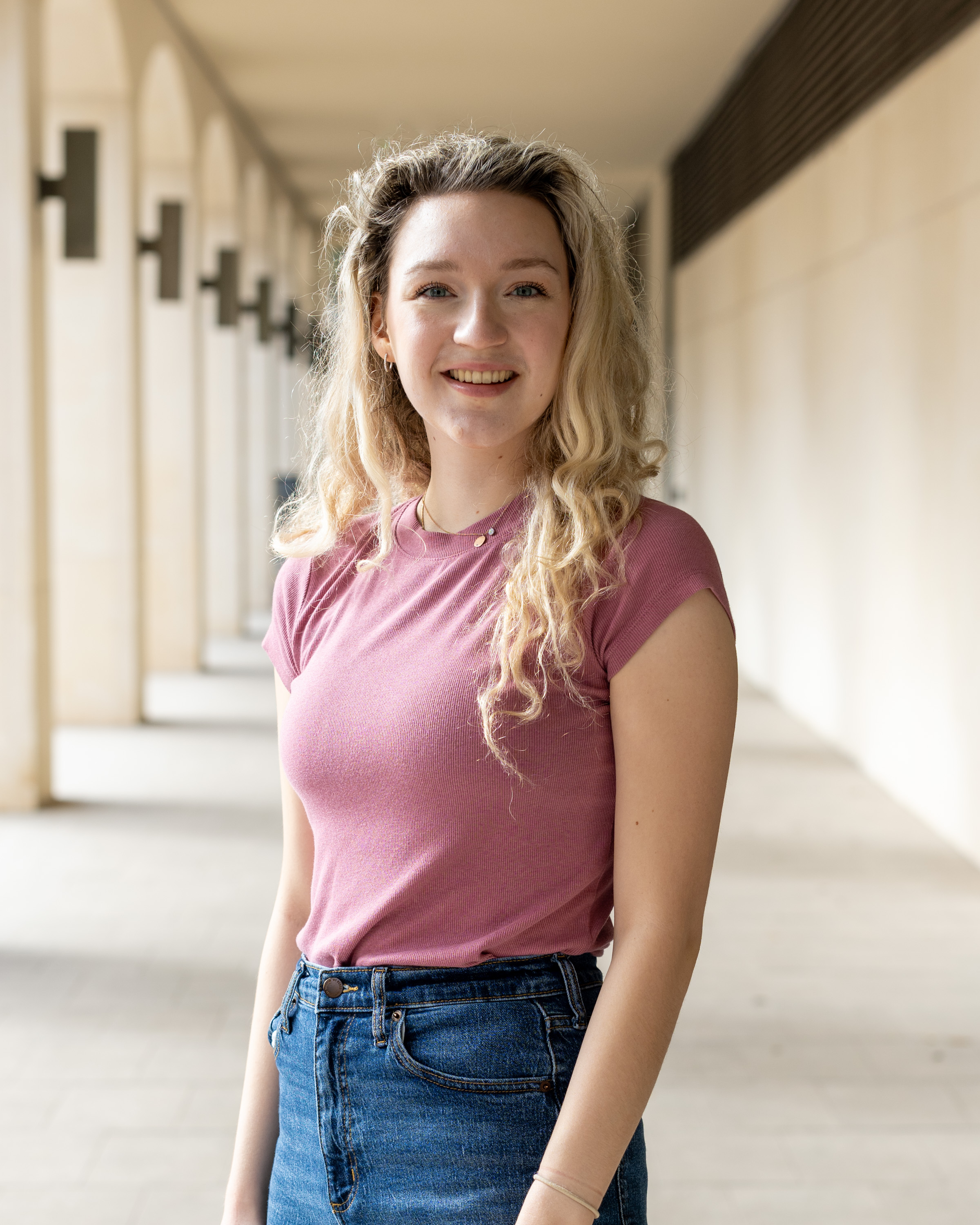
Jennifer Mickel
Towards Ethical AI
Inspired by the Netflix documentary “Coded Bias” about racial biases in facial recognition algorithms, Jennifer Mickel, a computer science fourth-year student, focuses her research on AI fairness. Biases in society are reflected within data, Mickel said, and a great deal of internet data represents a point of view centered on Western viewpoints.
“We don’t see as much representation from people who are not in the West, and so that means that the ideas of the people whose data we’re using to train our models are replicated within those systems,” Mickel said.
Improving fairness can involve using data and assessing an AI model’s performance in new and different ways. Much data is demographic-free due or has partial demographic information due to privacy or other barriers; nonetheless, many models perform better when given background information with demographics, Mickel said. That’s why Mickel works to improve models trained with demographic-free data. Using a system of finding intersections in data — and identifying and mitigating the effects of the worst-performing group — proves feasible for improving these models.
“Practitioners would use our algorithm to find the groups and then they would retrain using that group information to improve the performance for the worst-performing group,” Mickel said. “They would just keep doing that until the worst-performing group was performing as they had hoped.”
Early Starts
Besides all studying AI, Singhal, Padmanabhan, Sarma and Mickel have something else in common: their undergraduate research exposure started within their first year and a half at UT. In fact, the University has more first- and second-year college students doing real-world research than any place in the country.
Singhal said the early start afforded him the opportunity to learn his research interests and lead projects earlier in his research career. He also has formed strong connections with supportive lab members he’s able to ask for advice or learn from.
“I’m an NLP [natural language processing] researcher,” Singhal said. “I really love most of NLP. So it’s always been very exciting, and it keeps me motivated to keep on doing research.”