Live Show: AI + Energy
In our latest podcast, a panel of experts discuss the huge energy demands of AI and, on the flip side, how AI might make our energy systems better.
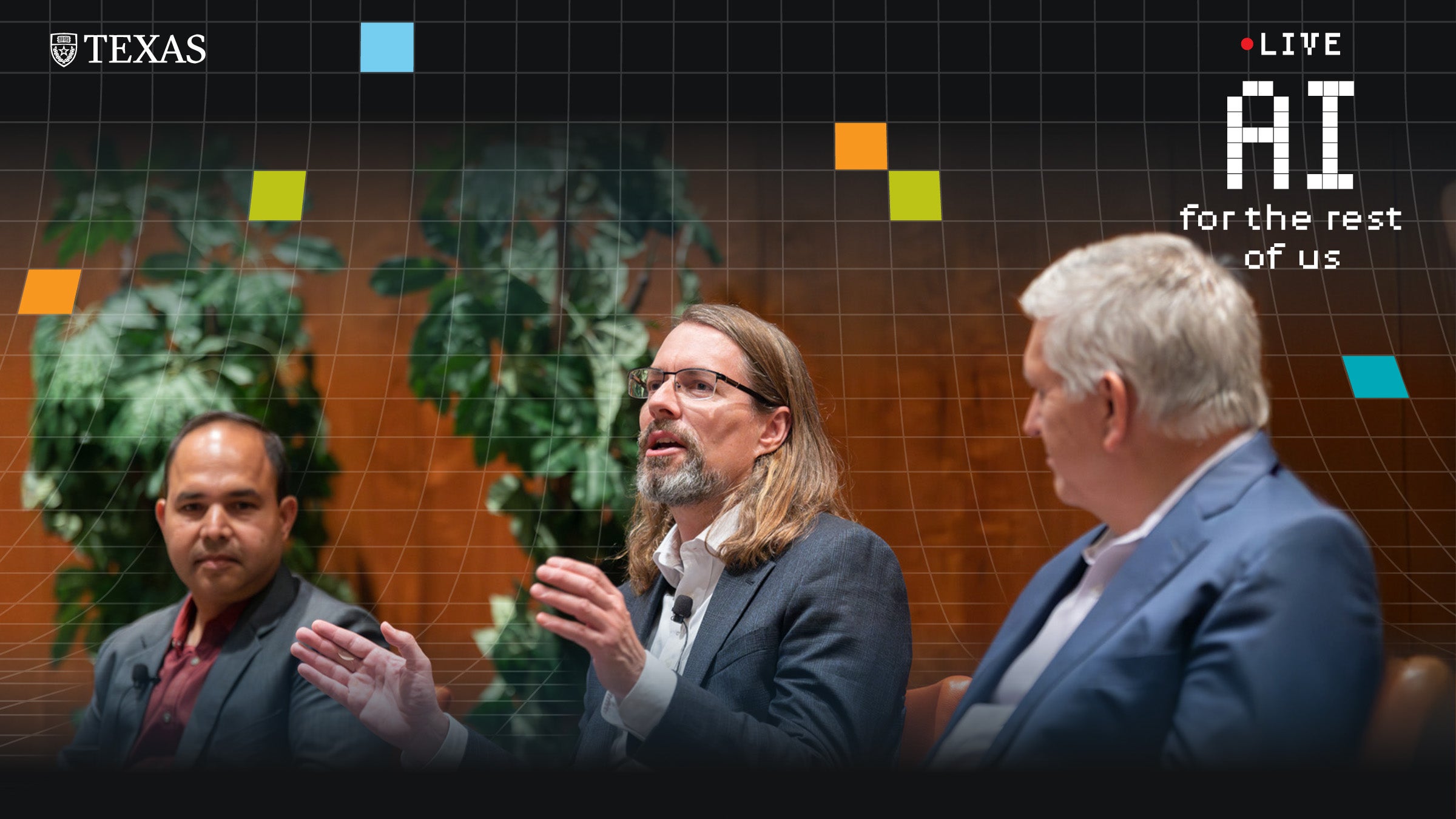
Photo by Thomas Meredith, courtesy of LBJ School of Public Affairs.
AI takes a huge amount of energy to run and could make it harder to fight climate change. On the other hand, AI could help make our energy systems more sustainable, efficient and safer. Three experts talk all things AI and energy with a live audience. The talk was part of a daylong symposium titled “Policy Leadership in the Age of AI”, hosted by the LBJ School of Public Affairs at The University of Texas at Austin.
Meet the panelists:
Michael Pyrcz is a professor in UT’s Cockrell School of Engineering and the Jackson School of Geosciences, who researches and teaches about ways to apply data analytics and machine learning to improve the exploration and safe production of minerals, groundwater and conventional energy, a.k.a. oil and gas. He also shares educational content on YouTube and elsewhere under the alias GeoStatsGuy.
Varun Rai is a professor in UT’s LBJ School, who studies the spread of clean energy technologies and how real-world factors – from economics to politics to regulation to social behaviors – drive the adoption of these technologies.
Rob James is an attorney at the law firm Pillsbury, who leads a number of energy and infrastructure projects for the firm in Texas and California. Those projects have included AI data centers and zero-emission power generation and storage.
Dig Deeper
The A.I. Power Grab, NYTimes (Oct. 2024)
A bottle of water per email: the hidden environmental costs of using AI chatbots, Washington Post (Sep. 2024)
Four ways AI is making the power grid faster and more resilient, MIT Technology Review (Nov. 2023)
Microsoft deal would reopen Three Mile Island nuclear plant to power AI, Washington Post (Sep. 2024)
Extreme Weather Is Taxing Utilities More Often. Can A.I. Help?, New York Times (Sep. 2024)
Fixing AI’s energy crisis, Nature (focused on reducing computer hardware’s power consumption - Oct. 2024)
A.I. Needs Copper. It Just Helped to Find Millions of Tons of It., New York Times (July 2024)
AI is poised to drive 160% increase in data center power demand, Goldman Sachs (May 2024)
Photos from Policy Leadership in the Age of AI Symposium (Oct. 2024)
Episode Credits
Our co-hosts are Marc Airhart, science writer and podcaster in the College of Natural Sciences and Casey Boyle, associate professor of rhetoric and director of UT’s Digital Writing & Research Lab.
Executive producers are Christine Sinatra and Dan Oppenheimer.
Sound design and audio editing by Robert Scaramuccia. Theme music is by Aiolos Rue. Interviews are recorded at the Liberal Arts ITS recording studio.
The cover photo for this episode is by Thomas Meredith, courtesy of LBJ School of Public Affairs.
TRANSCRIPT
Marc Airhart: To close out “AI for the Rest of Us,” we have another special episode. On October 1st, here on the UT campus, three energy experts joined me at a live event hosted by the LBJ School of Public Affairs. AI takes a HUGE amount of energy to run. Conversely, AI could help to make our energy systems BETTER: more sustainable, safer and efficient, too. We talked about all of that and more.
Casey Boyle: Joining Marc were two UT faculty members: Michael Pyrcz [perch], a professor in the Cockrell School of Engineering and the Jackson School of Geosciences, who researches and teaches about ways to apply data analytics and machine learning to improve the exploration and safe production of minerals, groundwater and conventional energy, like oil and gas. And Varun Rai, a professor in the LBJ School, who studies the spread of clean energy technologies and how real-world factors – from economics to politics to regulation to social behaviors – drive the adoption of these technologies. Joining them also was Rob James, an attorney at the law firm Pillsbury, who leads a number of energy and infrastructure projects for the firm in Texas and California. Those projects have included AI data centers and zero-emission power generation and storage.
MA: This interview has been edited for length and clarity. And now without further ado, here’s our conversation.
MA: Let's start off with talking here about our kind of first big bucket, which is about the energy use to run AI and Rob, maybe you could kick off. So you're an expert on energy infrastructure, and I wonder if you could talk just a little bit about how much energy AI uses and how fast that is expected to grow over the next few years. And maybe we could talk about both, sort of the national level, but also some of the things that might be unique to Texas as well.
Rob James: Great. Well, thanks, Marc. I'm very happy to be here. I did have to research this a bit, but it's daunting, the amount of energy consumed by Texas in general, and for AI in particular. The overall energy picture for Texas, is about 85 gigawatts. It's a lot of energy. That is expected to grow to 150 gigawatts by 2030, uh 5 or so years from now. The AI use of energy in Texas right now is a little under seven gigawatts. And that is projected to increase, in other words, of that increase that we're going to see by 2030, much of that is going to be through the expansion of the power and cooling needs of data centers in the state. So it's, it's a prodigious amount of energy already, and it's going to get bigger.
MA: So that's Texas, but and then the nationally, I guess we're going to also see large increases?
RJ: That's right, the projections are that as a nationwide use of AI, at the moment, it's about 3% of our energy bucket that's projected to go to 11 or 12% by that same time period, 2030, or 2032, so it's going to be a large segment of American growth as well.
MA: And so I'd like to kind of open up to anyone who wants to jump in on this. But you know, will our energy systems be able to keep up with this? You know, rapid rise in demand.
RJ: Well, we're already seeing stresses on the system in terms of the review and implementation of new data centers. To back up, data centers, originated in the tech corridors of Northern Virginia, Santa Clara Valley in California. But now data centers are going wherever they can find reliable energy, where they can find water for cooling or cold ambient temperature, and an entitlements process that's going to be able to site these facilities. And it's also competing against other uses for electric vehicles, for the electrification of society, more broadly, even for climate change projects like direct air capture that are quite prodigious energy users as well. So big tech and big green are somewhat in competition for the same resources, because the big tech companies are focused on zero emission, whether that's carbon capture with gas-fired power, renewables with storage or even nuclear or geothermal sources. So it's broadening the number of resources available, but it's showing that there are strains on the system of being able to accommodate the general run of data centers.
MA: Any other thoughts on that, like, will we actually be able to keep up? It sounds like maybe a little little skeptical, Rob, that enough data centers can be built fast enough and enough power can be mustered. Any other thoughts on that?
VR: Yeah, I think there are two other things. And I will spin the question a little bit. Do we really want to meet all the needs, given our current makeup of the energy system, right, with emissions and water use and so on, is that the same makeup that we are going to use? And obviously the answer is no. And so that frontier will and needs to change, importantly. And the second piece is the amount of energy and resources that are being used that itself will change. These processes, these algorithms, architectures and data centers, they will all become a lot more efficient. So this is a very, very fast, changing and dynamic scenario, and we'll need to keep that fabric in mind and only project what's there in the current time frame to a limited amount, and be open to asking, you know, these more complex, dynamic questions.
MA: And so Rob, you mentioned a little bit of some of the different options, the nuclear, renewables. And, you know, Varun, you also mentioned sort of efficiency. What, are there some other ways that we might be able to potentially overcome these, these bottlenecks in providing enough energy to keep all the AI wheels flying?
Michael Pyrcz: So this is the thing I know, is that Texas has been able to innovate when it comes to energy sources. You know, one thing that's kind of near and dear to my heart, I imagine, you know, our natural gas production and LNG that now is supplying Europe and really replacing and providing energy security for Europe. I think Texas has been just world class when it comes to developing energy sources. I don't know how many people know this, but the depths at which we're working in the Gulf of Mexico are incredible, and the types of challenges we have to get at those hydrocarbons, deep, deep, deep in the deep water is just really incredible, and it's all at every step, it's been a technology hurdle and a challenge, and then we were able to develop new types of technologies and solve those problems and unleash new energy sources. So I have some confidence when it comes to Texas.
VR: This raises a very important point, which is the way I think about AI in its current state, is that it's a double-edged sword. It has goods and it has bads. It can really speed up the innovation cycle, increase efficiency and productivity. It could also, if we don't use it mindfully and resource it mindfully, it could also accelerate climate change and hasten our path toward Doom, if you will, right? And the art is going to be, how do we double down on the goods? How do we incentivize and progress on the good, and how do we curtail the bad sides of things? So every time we say we are going to be able to fuel it, we as scholars and we as members of the society should also be very, very carefully and mindfully thinking, well, what are we producing? What are the impacts? It's not just about fueling AI. It's – At the end, it's about us. It's about our society and about this planet. What are we doing in doing that?
MA: I think that's an excellent point, right? It's, why are we doing this, right? So what's, uh who benefits from these technologies, and what are the risks and downsides? And I think that's a good segue into something else I wanted to ask, which is, there's a lot of concern now about how this rising energy use could accelerate climate change, right? If a lot of this energy is being produced in a way that adds carbon to the atmosphere, is it possible to feed this growing energy demand for AI without increasing carbon emissions?
RJ: Well, in the near term, I think that a lot of technologies are in deployment phases, and so there's going to be some catch up before you can really say that there's no impact from that. A lot of these proposals for nuclear, for advanced geothermal, are quite a ways off. And what you have right now is the paradox that by insisting on zero emission power for themselves, the data centers are requiring coal fired generation to stay online longer. That coal fired generation plants are being restarted to accommodate the drivers, and that projects involving climate change, like direct air capture, are having to suspend operations because they can't get the green power for their duty. So I think we are seeing these kinds of strains and interruptions in the progress towards goals that people had just three or four years ago, Marc, in terms of making greater contributions towards zero emission power. These things are going to come, but they're going to take time.
MA: Okay, so there may be some unintended consequences of ramping up this energy use. Is Michael, do you have some thoughts on that?
MP: So if you look at society, it's an extremely complicated, high dimensional problem. And if you think about it, the way we've been running it so far is pretty inefficient. You can look around yourself and see all kinds of inefficiencies. I have a student team of about 15 PhDs working with AI. And one of the big things we do is we take complicated systems and we do what we call dimensionality reduction. We reduce them to much simpler systems that we can understand and optimize. And I'm very confident that we're going to be able to develop a lot of great ways to optimize society so that we're more efficient with our energy use. I think that that will be a great savings and will help us protect the environment. But I want to, I don't want to be the negative person in the room, but I'm going to say it, all human activity has consequences. I think that's something really important that we can't really, there's no panacea solution which helps zero environmental consequence. No one's gonna disagree with me? I'm not in trouble?
VR: No, I actually want to take a step back and add a little bit more complexity to the issue you raised, but then, you know, add a very positive dimension to it. … anytime you or I are tempted to make use of AI, what is it that is driving us to do that? Doing something better, saving time, being more productive, something. There's a lot of heterogeneity in this world that is driving people, counties, states, countries, good and bad actors to be using AI. So, it's gonna get used. And if you fuel it using the sources that we have currently, it's gonna further accelerate the stresses on climate, Now let me add the very positive thing, which is what really excites me, and that's what I really study, the innovation system. And the idea is that AI can really speed up different parts of the innovation cycle, right? It takes a lot of time, a lot of iteration to go through this, lot of investment and energy of all things is actually very hard to innovate in, right? Because very capital intensive. It takes years and years and all the conventional models of venture capital and software industry, and you know, other parts, for example, in biotech and in drug discovery, those same models don't actually apply to energy R and D, but, but AI has that promise of really short circuiting all of that, leading to innovations that can address the very same issue with climate and resources and so on and so forth. So I think it goes back. We are at this crossroads, and we need to be very intentional to be going down this path of making these positives and benefiting from those, but also being intentional not to add on to the bad part. But as Michael said, you know, it's not as if you know we can never have any consequences. We'll have, we just have to manage them and be very intentional.
RJ: I think what all three of us are saying is that there's a balance sheet that you need to apply for both the energy benefits and energy consequences of the introduction of greater use of AI in our system. And we're to as attuned to the benefits and efficiencies as we are to the to the consequences and to the time periods in which you're going to have the incidence of these positive and negative factors.
MA: That makes a lot of sense, and that's actually a good segue into kind of our second big bucket of, basically, how AI itself can help make our energy systems better in various ways, whether it's producing more energy or doing it more safely or more efficiently, or, you know, with less environmental impact, that sort of thing. And maybe, Michael, maybe you could kick us off in this kind of second part. So you're sort of an expert on using AI in finding, you know, finding, finding and producing subsurface resources. So maybe you could talk a little bit about without going into super technical detail, but a little bit little bit more detail about what your work is focused on.
MP: Thanks, Marc. I hoped you were going to ask me so. And let me just say something I think that's important is that my team, we work on all subsurface resources, groundwater, oil and gas, of course, but also minerals and so as we're having this discussion here, I want everyone to remember that all of this energy transition requires a lot of new minerals, rare earth elements and so forth, that we need to mine. The solutions are often in the subsurface. That's what I like to tell my students. Okay, now the first thing I'm going to just make a couple of comments about AI. I get really excited because we're able to do things we only dreamed of doing decades ago. For instance, we can predict at high resolution, I mean nanometer, the flow of fluids going through the porous subsurface. We can see it flow. We can predict and forecast that a high accuracy, and we can scale that up to scales that are relevant for energy production. And to me, that's amazing. The other thing we can do with AI, it's fast, and so when we build these models, we can make a change in a development scenario and get instantaneous feedback. We can see real time the consequences of our engineering and geoscience choices on the environment, on production, on reliability. So these are really helping us. And what do I see all of this doing? If we better understand the subsurface, we can minimize our environmental impacts, we can maximize safety, and we can do a better job producing these resources that we need. And so I get really excited. This is all being done through AI. We can see much more, do much more.
RJ: I might add that above the surface, in renewable energy applications, AI is also finding ways for efficiency. We're seeing, for example, that wind turbines are usually oriented based on longer term wind patterns, but AI is now capable of detecting even small gusts and immediately changing the yaw and the pitch of wind turbines. And my favorite application above ground is that purchasing for renewable energy depends on when you expect peak energy usage to occur. In Switzerland, they started gauging how much energy was going to be used two hours after a traffic pattern. So they would detect traffic on the roads, detect how that was going to affect when people were going to be coming home and turning on the devices. And they were able to achieve energy savings in terms of efficient purchases of renewable and others and base load sources far better than what human planners were able to do by just integrating two seemingly unrelated factors. And we're probably going to see many examples of connecting the unconnected and producing greater efficiency in all this sector.
MA: That's fascinating. So using AI then to study patterns in human behavior then, and to help plan down the road for how much energy are we going to need in two hours, or …
RJ: However, there are some cautionary notes about how AI is used in energy. For example, AI is sometimes used to inform site selection, trying to figure out the optimal place for locating renewable energy assets or data centers and the like. And one factor they'll consider is real estate. And the AI will say, Well, this real estate over here is inexpensive, so let's go here. Well, why is that real estate inexpensive? Do you have an AI system that takes into account the environmental justice aspects of vulnerable communities already impacted by the lower value of the environment? … are you compounding existing biases in a system such as property valuation by incorporating it into energy decisions?
MP: For sure, one of the things that happens with AI is these systems can get very complicated. The models themselves, I had a student go to Los Alamos National Laboratory. They built a model. I said, Did you bring it back? “I didn't have anything big enough to carry it.” It was like that big. So just imagine, this isn't something you'll send in an email. And so these are models that are very hard to interrogate to understand what they're doing. And what can happen very quickly is that people start to trust the model. It becomes a system they can't interrogate it. It becomes an unquestioned authority. If I can give you an example, I had a student. They were all excited. They had used satellite information. They were able to automatically detect urban development. They were using it for mapping and seeing how cities grow, trying to figure out urban planning problems and challenges, things like water management, surface water runoff. And I went back to them, I said, Okay, what did you actually predict? Can you show me how your model would predict relative to pixel color? Student came back and said it was 100% correlation. Basically, they’d designed this very complicated model, and all it could do was on satellite photography, find grays and browns, tans, colors of buildings and roads. And so, you know, we have to be careful with these machines. We may not truly understand what they're doing and the biases, what we found is, if you have bias in your data and your training data, even if you test and apply it with unbiased data, that bias signal remains embedded in the model. So we have to be really careful about that. Thank you. I appreciate that.
MA: Is there anything you'd like to ask each other about in terms of this, this idea of using AI in the energy space?
MP: So one thing I'm really interested in is, if I look at the panel right now, there's representations that's not here, necessarily directly the industry, the people leadership in the industry are making kind of major choice and so forth. So I just wanted to ask my co panelists, how is it going as far as the partnership with industry? How are you finding them, their role as being part of the solutions and so forth? Would you be able to comment on that?
RJ: Well, I actually mentioned this in a class of Varun’s yesterday, is that somewhat refreshing to see a technology company's approach to energy project development, horizon and hurdle rates, that we have technology companies looking at investments that are not going to pencil out for a great period of time, that may not have a very high rate of return that would otherwise kill a project that is being pursued by an independent power producer funded by a an equity fund that is looking for an immediate return and immediate capture. Some of these technology companies see energy as an input towards what they forecast will be a much higher value of the end product of artificial intelligence, in particular, and their technologies more broadly. So that's one aspect that I found refreshing in all of this, is that a relaxed attitude towards the requisites for investment.
VR: And to add to that, you know, there is in the corporate world, there's a huge heterogeneity. A lot of the other companies, they're set up, you know to innovate in goods and services and be able to sell that and return a profit on that and be able to survive in the longer run. So most of the firms will look for what is the letter of the law. But again, it's a huge mix, where you're seeing these great leaders that are being very mindful of the moment, and others are like, hey, we need to be able to do better and innovate in our products and services. And AI can really help us. And let's move forward.
MA: Great. Well, I think this is good time to kind of shift into our third and final bucket where we talk about sort of societal, security and innovation aspects of AI, and we'll keep leaning on you a little bit, Varun, since this is kind of in your wheelhouse. Will all the rising demand for AI and the changes that makes in our energy system, is that going to cause us as private citizens to have to pay more for our electricity?
VR: Well, as Rob alluded to earlier, it could. It possibly could, depending on how the costs of transmission system are socialized, where exactly the peak in the system is being peak, low. That is the increase in load at the maximum usage is happening, how that all is priced, and so on. So it certainly could, up until some point. What we are also seeing is it is possible to develop parts of the system, for example, a lot of base load capacity that can be very useful, both in terms of generation, but then also in terms of other transmission, distribution assets that could be very helpful to the broader system in other parts of the day, of the year, but also, in particular in times of emergencies, right? We here in this state a little over three years ago, experienced URI and all the devastation that it did to our people and infrastructure. And there is a possible pathway here where, through development of this infrastructure thoughtfully, can actually be helpful, and if it is not done, it certainly can lead to cost increases for the masses.
MA: There's also been a lot of debate about safety and security risks with AI. Can you talk a little bit about that Varun about, sort of generally, and also with regards to energy, tell us a little bit about sort of the security concerns there.
VR: So this is, this is what is talked about as the negative side of AI, the safety parts are basically, you know, think about the gorilla problem, which is, we create a very super intelligent system, and that leads to our own extinction, right? And so that's, that's kind of the very, very extreme. And there, you know, they're thinkers that that do think about that. More to present the safety aspects include disinformation, misinformation, deep fakes, use of these systems by bad actors, state or non-state, for development and potential use of biological and chemical weapons and so on, so forth. That's kind of the broader realm of AI, safety and security, more specifically, to energy. I did allude to earlier as how, if we use and power and grow these systems, using the current state of the energy system, right, and how it interacts with climate change and our broader resource and sustainability aspects, that's gonna really ripple through and create other non-linearities, and will accelerate and take us down a very, very different path. That's where we need to be very, very careful and chart, of course, that is cognizant of this possibility.
RJ: One thing that I've seen in observing how individual data centers are run that I think probably extends to the whole energy infrastructure system, is the need for redundancy and for alternative routes. That most of the systems, power and cooling systems, have a fail-safe, that there are two generators, not one generator line coming in, that there are two different Uninterruptible Power Systems coming in to make sure that the system stays online. And so just as in other areas of kind of military strategy and diversity, is security, so that you don't have yourself entirely dependent on a single system that can have a point of vulnerability, whether through negligence or intentional acts, that you can focus on and bring down an entire system. It may be contrary to your goals of greater economic efficiency to have that redundancy, but I think that's an essential element of individual energy assets, and I see no reason why it wouldn't apply to the system as a whole.
MA: Do you feel like there should be some regulation around the amount of energy that's being used for AI, and how that would work, like, what's the, you know, is there a practical mechanism for for, you know, maybe controlling that without it affecting innovation and that sort of thing.
VR: When we think about AI with all these complex goods and bads. And it's so natural to think, hey, one way, great way of cutting off the bads is regulating and so on. We have to be very careful. Knee jerk regulation and policies are actually a very bad idea. I think of regulation in these complex scenarios as blowing fall leaves, and right now, leaves are falling. So I'm into that. You blow too hard, and you can't control the movement of the leaves. You can't get them to you where you want, and you blow too weak, the leaves won't move. Same thing with regulation, if a regulation has to be very well crafted and it has to be adequately powered, not overpowered, otherwise, you will in your effort to kill the bads, you will also kill the goods. These are all the large companies that we are talking about. These are all multinational firms. If you do piecemeal regulation in one state, in one country, they can adapt and shift their shape, move somewhere else and keep developing these technologies. And you know, these are very, very heterogeneous. So what this really means is, in addition to very careful, adequately powered regulation, it has to be transparency, stakeholders and shareholders and regulators and activists engaging on an ongoing basis. This is not one of those where you there, you can craft a set of regulations and that that will do it. This is a very complex and dynamic problem, and we need to be all added, and that is the only way we are going to move it in the direction we desire it to.
MA: So piecemeal approach is not the way to go?
VR: Not at all.
RJ: Whether or not you cap it, there's a competition going on that through electrification, through more EVs, through electric vehicles, through the climate change matters I described, there's competition for those electrons, even the zero-emission variety, so that whether or not you're putting an artificial cap on it, there's a competition that's going on. So whether or not you regulate the energy needs for AI, it's regulated with a lower case r, it's going to be affected by these competing uses.
MP: The other thing when it comes to regulation is, as a graduate student, I was inspired by the book by Neil Postman, Technopoly. It kind of changed my life, and it basically taught me that technology has huge impacts on society. And I know that's axiomatic, of course, but one of the quotes in the book is that technology, once it's developed, it will play out its hand, and it's very difficult to put it back in the box. It's going to expand and find all kinds of uses, and it can become, it can be out of our control. And boy, I'm very scary today. Sorry. I'm a negative professor.
MA: I'm curious as kind of a final thought, given all the pros and cons we've talked about with AI and energy use, how should we proceed with using AI in the energy space. What's, what's your best advice for how we move forward?
MP: Okay, so, so I am developing technology to support energy extraction from the subsurface, minerals extraction from the subsurface, and this is what I know: we can build models we could never even dream of building before, with greater accuracy, greater precision, greater resolution. That's helping us develop the subsurface in a way that we can minimize environmental impacts, maximize safety in the extraction. So what I say when it comes to energy, keep going, but it's good. We've been doing data science, AI methods for a long time. We're going to keep doing it. We're going to keep leading the way, and I think it's going to help society, that's for sure.
RJ: Well my suggestion is, Rahm Emanuel’s “Never let a crisis go to waste,” which maybe this isn't a crisis, but it's certainly an opportunity. Don't let an opportunity go to waste when you have this generational demand for energy going up. Take the opportunity to make the leaps in investment required for advanced geothermal, for nuclear fission, even nuclear fusion. Take those steps that are needed because you now have people with a long enough time horizon, an ability to regularize and automate some processes that were not well managed. In the past, you've been given the opportunity of a very large quantum of demand from people who have a long time horizon. Don't let that go to waste by building more of the same. Use it to build something new and build something that lasts.
VR: Something I've learned recently from my own research is that crises can also lead to retrenchment, which is going back to the same bad ideas. AI has great promise here in the world of energy, as I alluded to earlier, energy innovation has been a very hard problem, really, if you have a big problem, you want to steer the ship, long time horizons, lots of capital. AI really helps with a lot of that. And so to the extent we can push on that frontier, we can address a lot of the concerns that we have been talking about. And that's my, both my hope and as well as effort in my own research too.
MA: Fantastic. That's a great way to wrap up that part of the conversation. Let's open it up the floor now. And if you will pass around the microphone, and if you'll say your name just briefly, who you are, what your connection to energy is, and what your question is, and just keep it brief so we can get through as many questions as possible.
Hasib Abdullah: Hello. My name is Hasib Abdullah. I'm a resident here in Austin. Fracking really changed the game with oil and gas here in Texas and across the world now, horizontal drilling changed the game with hydrogeology. Do you think AI will have the same impact? Thank you.
MP: So what do they say, like “AI is the new fire?” Is that what the tech people say? Something like that, right? “The new electricity” or something? Yeah, yes, yes. And so how do I say that confidently? It's because we've been using AI type technologies to invert and figure out what is in the subsurface given all kinds of complicated signals, and we've been doing that for decades. And now what we can do, I have a student who's doing Pix the Video. Have you all heard of that? I'll give you, give me a picture of a waterfall, and I'll run it through AI, and the water will flow. Give me a picture of fire, and it will start to burn. And we're literally using these types of technologies to predict the flow of heat and fluids in the subsurface. These are real game changers. And I do think, I would put it at the same level as these other technologies like directional drilling, yes,
RK: And I would say also the analogy is very apt, because the technologies for fracking were available sometime before. It was only the confluence of different types of technologies that brought it to the forefront. Similarly, I think it was the increase in processing speed and the ability to harness massive amounts of data that really transformed things from Alan Turing and Vannevar Bush and Claude Shannon, who may have thought of these things but never had the processing speed or the memory capacity to use them.
VR: I'll actually go a step further. One useful way of thinking about AIs is what are known as General Purpose technologies, technologies that are so foundational that they not only improve and build, but if they improve, that leads to improvement of lots of other things across the entire socio-technical system. We have been talking about energy, think about biology, think about medical sciences, you know, think about transportation systems, think about building systems, right? These are all things where AI really is is already starting to have and will continue to have. So in many ways, if you cut across the energy dimension, the answer is yes to your question. But you know, there are many similar dimensions like that, where AI is also going to be very powerful.
MP: I want to add to that. In addition, the technologies we're developing within energy are often used in many other sectors of our economy, and I think that's really interesting that, and I know this firsthand because other companies, tech keep hiring my PhD students.
Benjamin Rode: Okay, Benjamin Rode, Austin resident, long time researcher in formal knowledge representation and management. I want to ask you guys for a gut check I want to pose it in terms of this metaphor of the balance sheet, on the one hand, you've all talked about the tremendous potential inherent in AI for understanding complex systems, particularly in the in the energy sector, provide building efficiencies, optimization, discoveries in terms of understanding and mitigating the consequences of anthropogenic climate change, et cetera, et cetera. At the same time, the costs of pre-training and use are very, very high. You know, the carbon footprint, is not small. Do you believe that AI can get to the point where it is, so to speak, paying for itself in terms of this budget?
RJ: I think that is a great fundamental question, and it applies to subsidiary parts of what we all work on. I just tried to do that balance sheet, just in terms of whether we could be balancing the greater efficiency of the upgrades in in generative AI, which, as we know, is just one piece of it. But I had been hopeful. I had been thinking that the, you know, preparing the 1,000,000th high school book report on the Scarlet Letter would be cheaper than the first report on the scarlet letter. But I'm told that something like GPT 4.0 actually does more crunching on the prompt end of things, in addition to the training, so that some of that efficiency of a learning curve is being overcome by the fact that the higher upgrades are taking advantage of greater computation at the point of making the prompt and making the inquiry, so that blew my budget for what I was looking at. And I'm sure the thing is, the same thing is happening across all aspects of AI, where there's a balance sheet of benefit versus the cost. I would only say that there's a time element to all of this, and that there may be an initial investment that has to be recouped over time, and so you have to use the tools of financial analysis and discounts to really come to a nuanced answer to this excellent question.
VR: I think there are two balance sheets here, one of the firms that we are talking about, and I and others are probably cool. Many of these companies doing their investment with potential very large returns, and some of them will go bust and bankrupt. And that's the natural way of how, you know, we have set things up. The other balance sheet, which is where a lot of the downsides that we have talked about, in terms of climate, in terms of other safety, security, that's the balance sheet of the society. And that is where we need as a society, working with our and directing our government to direct the use of AI in all those positive areas, to double down, as I said, in sandwiching, fast tracking the innovation that is required and necessary and possible, as I said, the AI to really address those problems.
MA: All right, well, I'm afraid we're out of time, And with that, I want to thank you all as an audience for asking great questions and staying engaged. And would you help me thank our great panelists for their conversation.
About AI for the Rest of Us
AI for the Rest of Us is a joint production of The University of Texas at Austin’s College of Natural Sciences and College of Liberal Arts. This podcast is part of the University’s Year of AI initiative. The opinions expressed in this podcast represent the views of the hosts and guests, and not of The University of Texas at Austin. Listen via Apple Podcasts, Spotify, Amazon Podcasts, RSS, or anywhere you get your podcasts. You can also listen on the web at aifortherest.net. Have questions or comments? Contact: mairhart[AT]austin.utexas.edu